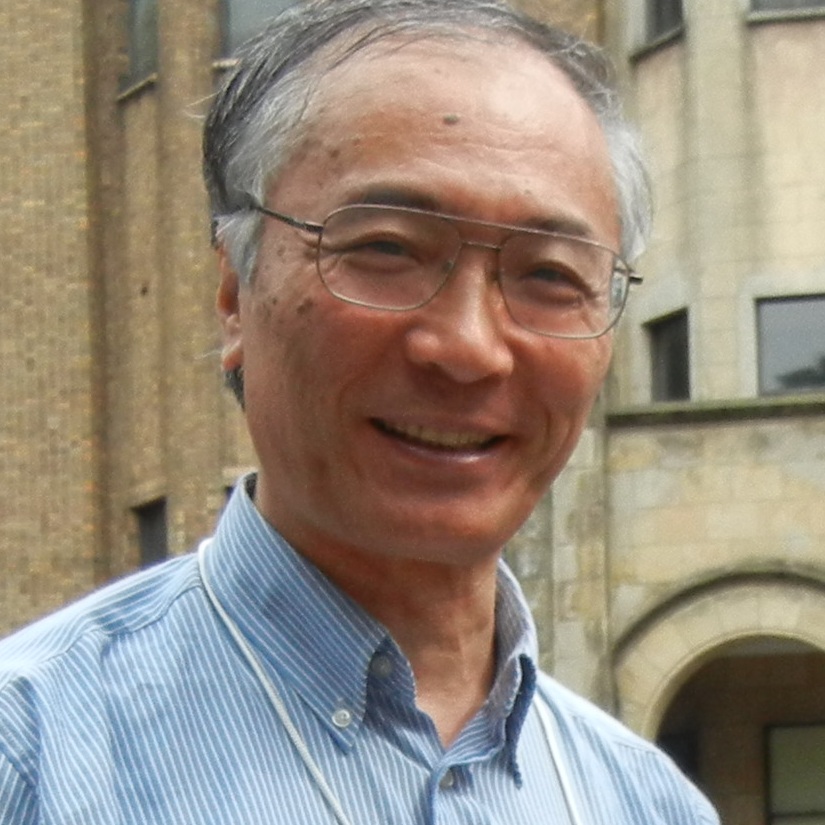
Shigeki Sagayama
Professor Emeritus, Graduate School of Information Science and Technology, The University of Tokyo Visiting Researcher, Graduate School of Informatics and Engineering, The University of Electro-Communications
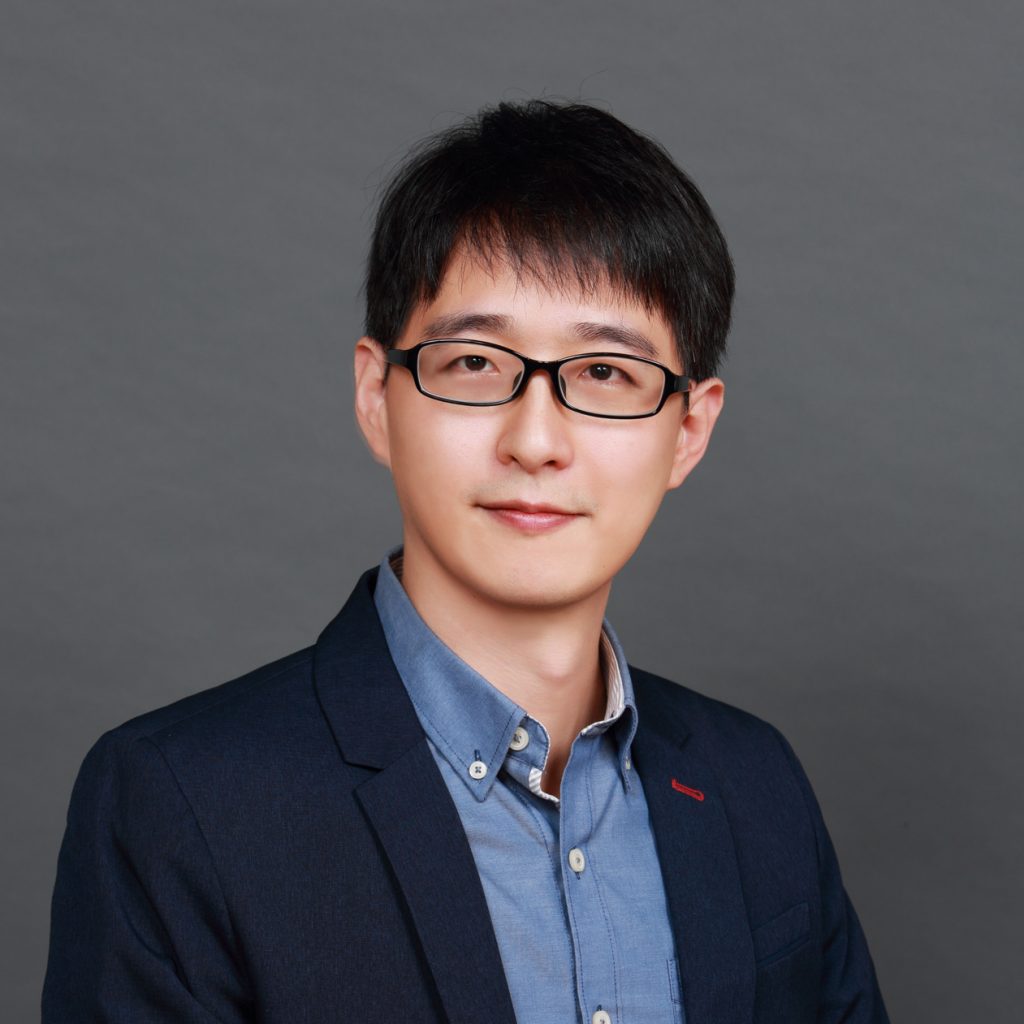
Yi-Hsuan Yang
Full professor, College of Electrical Engineering and Computer Science, National Taiwan University
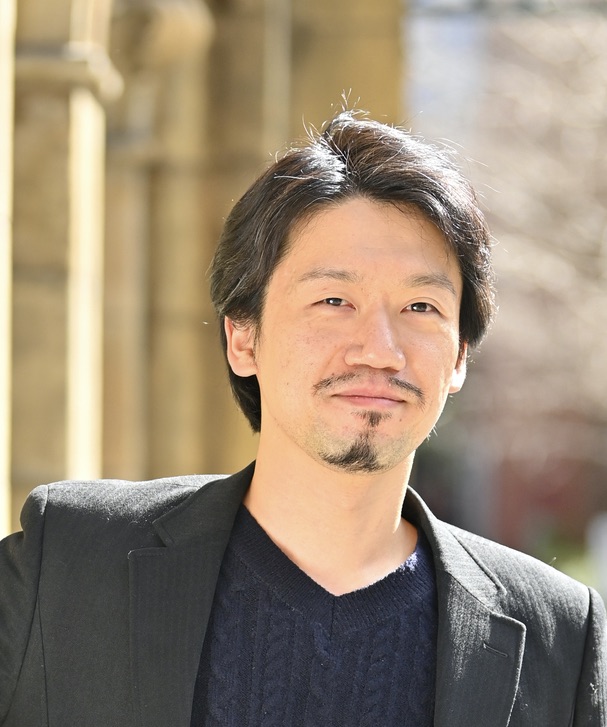
Tatsuya Daikoku
Graduate School of Information Science and Technology, The University of Tokyo
Lecture Title
17 Years with Automatic Music Composition System “Orpheus”
Lecture Title
Deep Learning-based Automatic Music Generation: An Overview
Lecture Title
Exploring the Neural and Computational Basis of Statistical Learning in the Brain to Unravel Musical Creativity and Cognitive Individuality
15, November (Wed), 15:00-16:00, Main hall
14, November (Tue), 15:00-16:00, Main hall
16, November (Thu), 15:00-16:00, Main hall
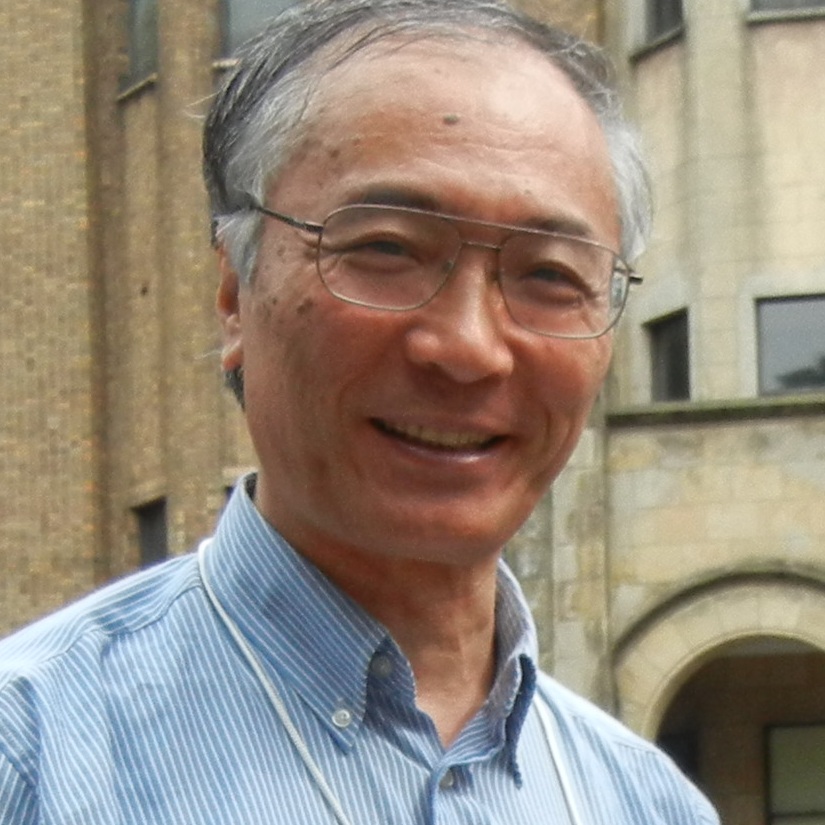
Dr. Shigeki Sagayama received the B.E., M.E., and Ph.D. degrees from the University of Tokyo, Tokyo, Japan, in 1972, 1974, and 1998, respectively, all in mathematical engineering and information physics. He joined Nippon Telegraph and Telephone Public Corporation (currently, NTT) in 1974 and started his career in speech analysis, synthesis, and recognition at NTT Laboratories, Musashino, Japan. He led an automatic speech translation project from 1990 to 1993 at ATR Interpreting Telephony Laboratories, Kyoto, Japan. After being responsible for speech recognition, synthesis, and dialog systems at NTT Human Interface Laboratories, Yokosuka, Japan till 1998, he moved to Japan Advanced Institute of Science and Technology (JAIST), Ishikawa, Japan where he started music processing research. In 2000, he was appointed Professor, the University of Tokyo (UT), Tokyo, Japan. From 2014 to 2019, he was with Meiji University, Tokyo, Japan. His major research Interests include processing and recognition of speech, music, acoustic signals, handwriting, and images. Prof. Sagayama is a Fellow of IEICEJ, a life member of IEEE, and received the National Invention Award from the Institute of Invention of Japan in 1991, the Chief Official’s award for Research Achievement from the Science and Technology Agency of Japan in 1996, and other academic awards including Paper Awards from the Institute of Electronics, Information, and Communications Engineers (IEICEJ) Japan, in 1996 and from the Information Processing Society of Japan (IPSJ) in 1995, and Achievement Award from Acoustical Society of Japan in 2021.
Lecture Title: 17 Years with Automatic Music Composition System “Orpheus”
Our research on automatic music composition was started at the University of Tokyo in 2006 after a long experience in speech recognition and synthesis, music processing, and other related areas.
Naturally, we took the probabilistic model approach toward computational melody generation to directly reflect the music-theoretic and linguistic knowledge and requirements rather than the machine learning approach to avoid collecting a enormous training data for imitating existing music pieces. To simulate well-trained human composers hardly violating music rules, we formulated melody generation as finding the safest path of state transitions in a Hidden Markov Model of time and pitch of notes so that resulted melody along the path best satisfies musical and linguistical probabilistic constraints and user’s preference while wide variety of outcome is guaranteed within correctness in academic music criteria. Model probability is empirically defined as the appropriateness mainly based on music theory of harmony and pitch-accent prosodic rules of Japanese language, and the optimal (i.e., least problematic) path is efficiently derived by a modified Viterbi algorithm similarly to speech recognition.
The current web-based version (“Orpheus” ver 3) for Japanese lyrics (https://www.orpheus-music.org/) was launched in 2012 along with the duet generation and voice and accompaniment sounding functions and became one of most popular music composition services that created 0.7M music pieces and received 19M access count through the internet during recent 4 years and has been often introduced by media (TV, radio, net news, newspapers, books, etc.) to the public as an example of generative AI.
Our 11-year experience of web-based service led us to further issues. To think of the universal melody generation model across both stress/pitch-accent languages (and hopefully tone languages in addition), 2-dimensional HMM is discussed instead of out current rhythm-tree approach. To truly assist the user’s creativity and to enhance their composition skills, we discuss the user interface for automatic composition as a composer’s workbench. Also, automatic music interpolation is discussed for mid-skilled users in music composition to interactively complete the music piece from fragments of melody, sub-melody and harmonies provided by the user.
Music (particularly, highly theoretically and academically sophisticated European classical music) can be positioned anywhere between the pair of extremes of artificial intelligence such as correctness-first math formula processing and eloquence-first natural language processing to consider the future research direction (possibly, a mixture of both).
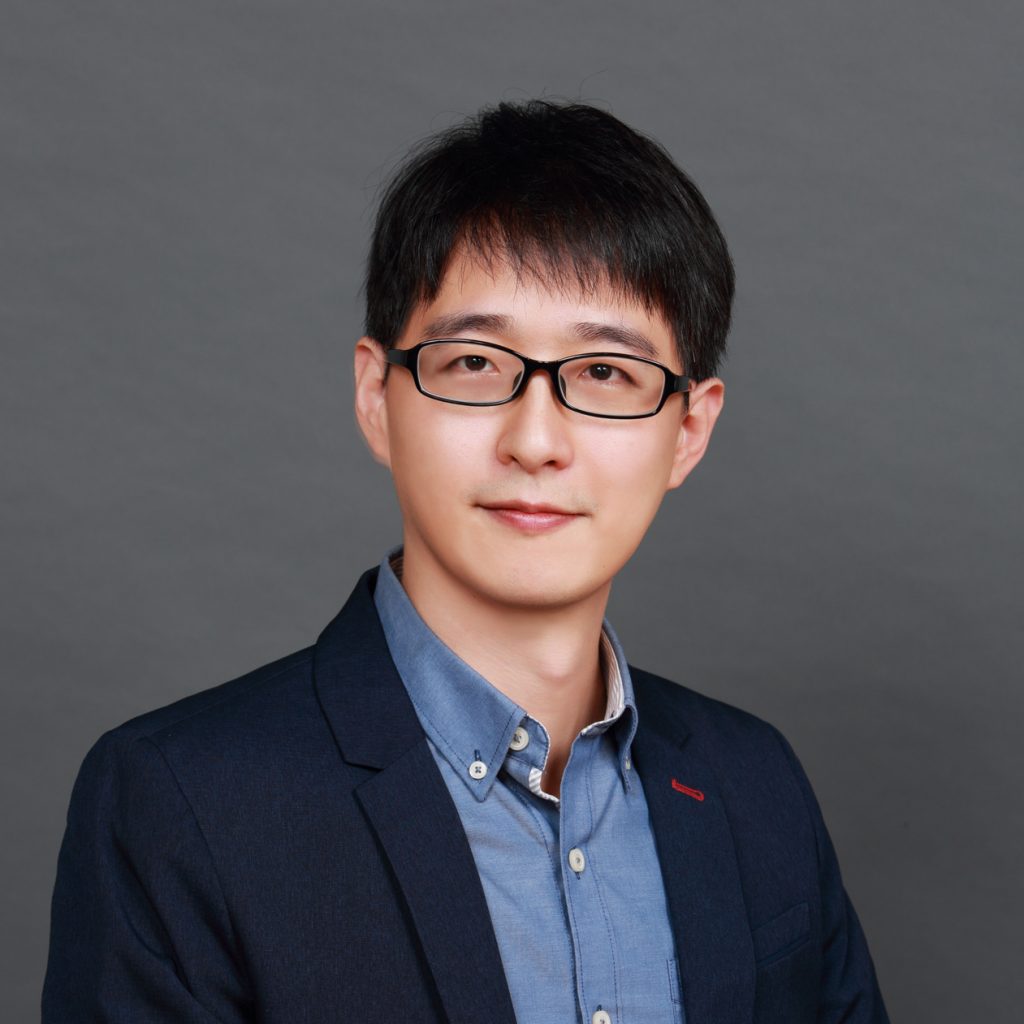
Dr. Yi-Hsuan Yang received the Ph.D. degree in Communication Engineering from National Taiwan University. Since February 2023, he has been with the College of Electrical Engineering and Computer Science, National Taiwan University, where he is a Full Professor. Prior to that, he used to be the Chief Music Scientist in an industrial lab called Taiwan AI Labs from 2019 to 2023, and an Associate/Assistant Research Fellow of the Research Center for IT Innovation, Academia Sinica, from 2011 to 2023. His research interests include automatic music generation, music information retrieval, artificial intelligence, and machine learning. His team developed music generation models such as MidiNet, MuseGAN, Pop Music Transformer, KaraSinger, and MuseMorphose. He was an Associate Editor for the IEEE Transactions on Multimedia and IEEE Transactions on Affective Computing, both from 2016 to 2019. Dr. Yang is a senior member of the IEEE.
Lecture Title: Deep Learning-based Automatic Music Generation: An Overview
This talk aims to provide a tutorial-like overview of the recent advances in deep generative models for automatic music generation. The talk has four parts.
In the first part, I will briefly mention data representations for symbolic-domain and audio-domain music that have been employed by deep generative models.
In the second part, I will use MIDI music generation to demonstrate the use of sequence models such as the Transformers to build the language model (LM) for symbolic-domain music, with a special focus on the modeling of the long-range temporal dependency of musical events.
In the third part, moving forward to the audio domain, I will review advances in timbre synthesis, generative source separation, Mel-vocoders, and audio codec models, to demonstrate the development of audio encoders and decoders for music, capable of generating short audio excerpts of music with high fidelity and perceptual quality.
In the final part, I will talk about how we can build upon technologies developed in the previous parts to create LMs for audio-domain music, and their applications to singing voice generation, accompaniment generation, as well as text-to-music in general.
I will conclude the talk with a few open challenges in the field.
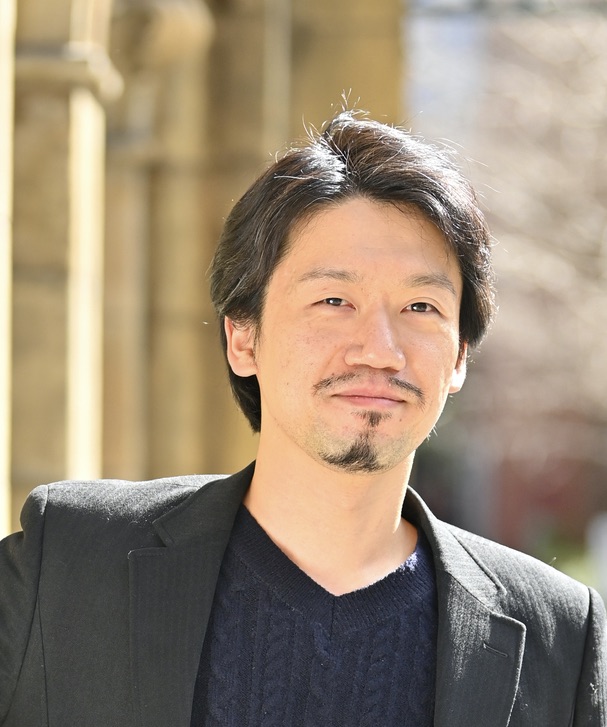
Dr. Daikoku is currently a project assistant professor at the University of Tokyo. He received his Ph.D. in Medicine from the same institution and has been a postdoctoral researcher at the University of Oxford, Max Planck Institute, and the University of Cambridge. Dr. Daikoku’s research interests lie in the interdisciplinary field of human and artificial intelligence, with a focus on creativity. Specifically, he endeavours to develop a computational model of creativity in the brain using neurophysiological data and understand the origin of creativity and its developmental process. Then, using the model, He is trying to generate a novel music theory that covers both computational and neural phenomena and compose contemporary music.
Lecture Title: Exploring the Neural and Computational Basis of Statistical Learning in the Brain to Unravel Musical Creativity and Cognitive Individuality
Music is ubiquitous in human culture. The interaction between music and the human brain engages various neural mechanisms that underlie learning, action, and creativity. Recent studies have suggested that “statistical learning” plays a significant role in musical creativity as well as musical acquisition. Statistical learning is an innate and implicit function of the human brain that is closely linked to brain development and the emergence of individuality. It begins early in life and plays a critical role in the creation and comprehension of music. Over time, the brain updates and constructs statistical models, with the model’s individuality changing based on the type and degree of stimulation received. However, the detailed mechanisms underlying this process are unknown.
In this talk, I will present a series of my “neural” and “computational” studies on how creativity emerges within the framework of statistical learning in the brain. Based on these interdisciplinary findings, I propose two core factors of musical creativity, including the critical insight into cognitive individuality through “reliability” of prediction and the construction of information “hierarchy” through chunking. Then, I will also introduce a neuro-inspired Hierarchical Bayesian Statistical Learning model (HBSL) that takes into account both reliability and hierarchy, mimicking the statistical learning processes of the brain. Using this model, I will demonstrate a newly devised system that visualizes the individuality of musical creativity. This study has the potential to shed light on the underlying factors that contribute to the heterogeneous nature of the innate ability of statistical learning, as well as the paradoxical phenomenon in which individuals with certain cognitive traits that impede specific types of perceptual abilities exhibit superior performance in creative contexts.